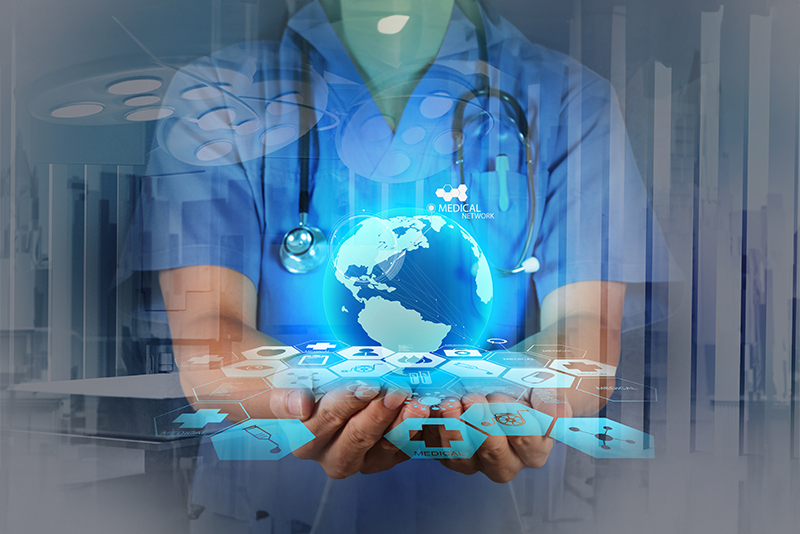
The advent of artificial intelligence (AI) has brought forth a new era in healthcare, promising improved patient outcomes and operational efficiency. However, the integration of AI into existing healthcare systems is not without its challenges. This paper explores the limitations of legacy systems in supporting AI applications and discusses the need for modern, AI-enabled systems in healthcare.
Artificial intelligence has emerged as a transformative force in healthcare, with applications ranging from predictive analytics to precision medicine. However, the integration of AI into existing healthcare systems, particularly those that are home-based, is often hindered by the limitations of legacy systems. These outdated systems, which were not designed with the capabilities to support AI applications, often lack the flexibility and scalability required to effectively integrate AI.
The Limitations of Legacy Systems
Legacy systems in healthcare are often characterized by siloed data and a lack of interoperability. This makes it difficult to aggregate and analyze data in a way that's conducive to AI applications.
Legacy systems also often struggle with the integration of AI due to their rigid and outdated infrastructure. They were not designed with the capabilities to support the complex algorithms and large data sets that AI applications often require. This can lead to inefficiencies, inaccuracies, and a lack of real-time insights, all of which can hinder the potential benefits of AI in healthcare.
The Need for AI-Enabled Systems
In contrast, modern, AI-enabled systems are designed with the flexibility and scalability required to support AI applications. They are built with advanced data analytics capabilities, enabling healthcare providers to leverage the power of AI to derive actionable insights from their data. AI-enabled systems also support interoperability, allowing for seamless data exchange between different systems and platforms. This is crucial for AI applications, as it enables the aggregation and analysis of data from various sources, enhancing the accuracy and reliability of AI predictions and recommendations.
Moreover, AI-enabled systems are equipped with advanced security features to protect sensitive data, ensuring compliance with healthcare regulations and maintaining patient trust. They also offer the ability to adapt and evolve with the rapidly changing landscape of AI, ensuring that healthcare providers can continue to leverage the benefits of AI as it continues to advance.
Conclusion
The limitations of legacy systems pose significant challenges to the integration of AI in healthcare. However, the transition to modern, AI-enabled systems can overcome these challenges and unlock the full potential of AI in healthcare. As we navigate the complexities of modern healthcare, the adoption of AI-enabled systems is not just an option—it's a necessity.